Language is increasingly being used as a diagnostic tool in biomedical research and has recently begun to be leveraged in psychedelic research. It turns out analysing language through machine learning can help increase diagnostic accuracy and predict psychedelic treatment outcomes, which will play an important role in the future of psychedelic research.
Illustration: Anna Temczuk
ㅤ
Language as a diagnostic tool
Sigmund Freud and Carl Jung are arguably the most influential figures of the 20th century when it comes to psychological functioning and the human mind. Although their theories about the psyche eventually differed, they both considered language as a manifestation of the unconscious. Indeed, Freudian psychoanalysis proposed free association as a way of gaining access to unconscious processes, while Jungian psychology considered every act of speech as a psychic event, with each word carrying particular archetypal energies. Fast forward 100 years, innovations in biomedical science and technology have transformed language into a diagnostic tool for both affective and degenerative neuropathology, and language is increasingly being used as such in psychedelic research.
Natural Language Processing, also known as NLP, is a field combining linguistics, computer science, and artificial intelligence. It applies computational techniques to the analysis and synthesis of natural language. One of the problems with natural language is that it often contains ambiguities in meaning, also known as semantic ambiguities, which are easily detectable by humans but not so much by computers. Luckily, models such as distributional semantics, count vectorisation and encoder-decoder modeling help decipher semantic ambiguities. Since its development, NLP has predominantly been used as an automation tool for google searches, spam email categorisation, voice recognition, and translations, but it is increasingly being used as a diagnostic tool in medicine.
A few years ago, a team of researchers in Canada were able to identify linguistic features within narrative speech that were specific to Alzheimer’s Disease. Semantic impairment, acoustic abnormality, and syntactic impairment were all factors enabling the accurate identification of Alzheimer’s, based on patients’ short descriptions of a picture.
This led to the realisation that beyond its unconscious, psyche-revealing properties, natural language might also possess neuropathology-revealing properties. So what if language could be used as a biomarker for psychosis or affective disorders? More importantly, what if language could be used as a predictor of treatment outcome? It turns out these tools have already begun to be leveraged in psychedelic research.
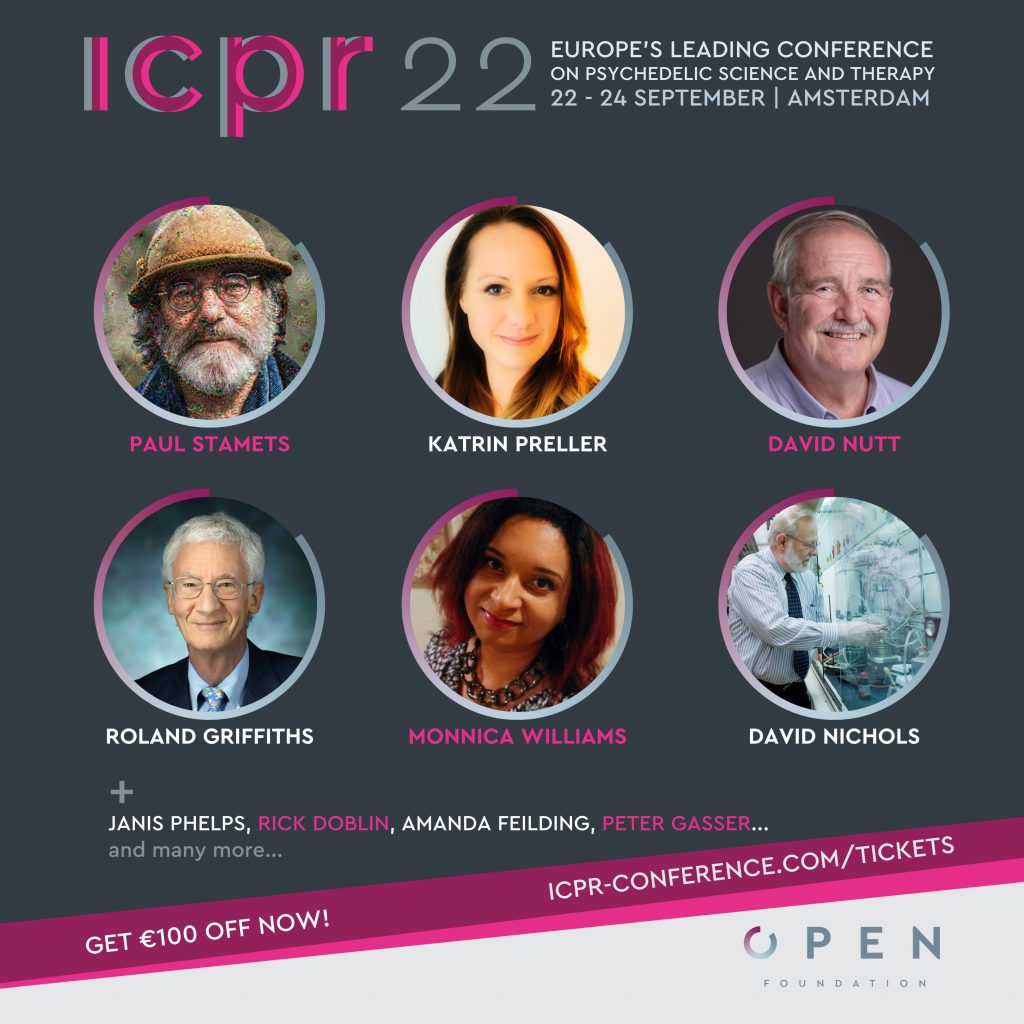
Around four years ago, a team of researchers from Buenos Aires University’s Applied Artificial Intelligence Lab and Imperial College London’s Psychedelic Research Group decided to test a combination of NLP and machine learning. They tested this combination both as a diagnostic tool for patients suffering from treatment-resistant depression, and as a predictor of treatment outcome following a psilocybin challenge.
Participants first underwent a psychological interview known as an Autobiographical Memory Test, an interview used to assess the degree of specificity of autobiographical memory. This interview was analysed using an NLP method known as Emotional Analysis, which quantifies the emotional content of spoken or written text. The NLP output was then fed as input into a machine learning algorithm, known as a classifier, trained at recognising depressed patients.
On the basis of emotional analysis and specifically the use of positive words, which were less frequently used in depressed patients compared to healthy controls, the classifier was able to differentiate between depressed patients and healthy controls with a mean accuracy of 82.85%, close to 15% better than the mean accuracy of general practitioners unassisted by screening tests.
Accurate Predictions
Perhaps more impressive than its ability to differentiate between depressed patients and healthy controls, was the classifier’s ability to differentiate between treatment responders and non-responders. Based on the same parameters it had previously used to diagnose depressed patients (NLP output and positive word frequency), the classifier was able to predict which patients would respond to a psilocybin challenge and which would not.
Only the patients identified as “responders” were given the psilocybin challenge, whereas the “non-responders” were removed from the treatment arm. This manoeuvre had the effect of improving overall treatment response by 34% compared to the original experiment.
Last year, a team at Johns Hopkins University used a similar approach to predict changes in substance use following a psychedelic challenge. They recruited individuals who reported quitting or reducing a number of addictive drugs following a psychedelic experience, and asked them for a verbal narrative of the experience.
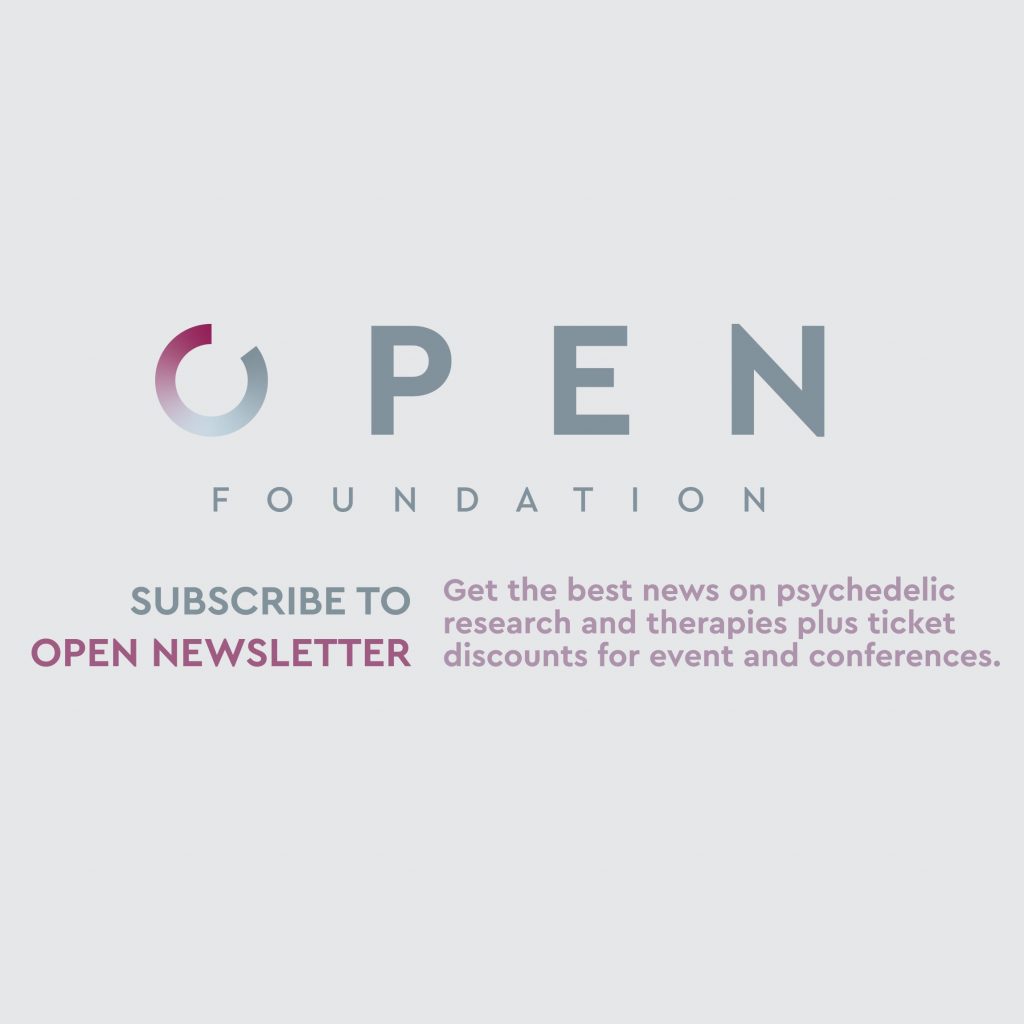
They used an NLP method known as Latent Semantic Analysis, which analyses the relationship between semantic structures across different texts, to derive topic models that described the psychedelic narratives. These topic models were fed as input into three different machine learning algorithms to predict long-term drug reduction. The machine learning algorithms had an average predictive accuracy of 65%, and additional analyses revealed between-group differences in psychedelic experience narratives based on the derived topic models.
John Hopkins’ semantic analysis of psychedelic narratives and Buenos Aires University’s use of machine learning to identify patients suffering from depression, are two early but powerful examples of the ways in which language can be leveraged in psychedelic research through new technology.
The combination of NLP and machine learning as methods to analyse language have reliably shown their value as both diagnostic and predictive tools, and can be used to optimise clinical trials. They allow for a more personalised treatment, whereby non-responders are spared the emotional rollercoaster of an acute psychedelic experience.
Freudian psychoanalysis, Jungian psychology and NLP share the conception that hidden semantic structures within language are associated with underlying processes, whether psychological, social, or physiological. A century ago, language was the glass through which Freud saw the unconscious mind. Today, language analysed by machine learning may very well be one of the prisms through which we can come to understand the psychedelic experience.
ㅤ
References:
1. N.B. This is different from “Neuro-linguistic programming” (NLP), which is a form of psychotherapy developed in California in the 1970s, mainly used as a method of personal development by promoting skills including communication.
2. Fraser, K. C., Meltzer, J. A., & Rudzicz, F. (2016). Linguistic Features Identify Alzheimer’s Disease in Narrative Speech. Journal of Alzheimer’s disease : JAD, 49(2), 407–422
3. Carrillo, F., Sigman, M., Fernández Slezak, D., Ashton, P., Fitzgerald, L., Stroud, J., Nutt, D. J., & Carhart-Harris, R. L. (2018). Natural speech algorithm applied to baseline interview data can predict which patients will respond to psilocybin for treatment-resistant depression. Journal of affective disorders, 230, 84–86
4. Carey, M., Jones, K., Meadows, G., Sanson-Fisher, R., D’Este, C., Inder, K., Yoong, S. L., & Russell, G. (2014). Accuracy of general practitioner unassisted detection of depression. The Australian and New Zealand journal of psychiatry, 48(6), 571–578.
5. The original experiment consisted of a combination of psychotherapy and pharmacological treatment with psilocybin that resulted in 41% treatment response. By differentiating between treatment responders and non-responders this experiment resulted in 75% treatment response
6. Cox, D. J., Garcia-Romeu, A., & Johnson, M. W. (2021). Predicting changes in substance use following psychedelic experiences: natural language processing of psychedelic session narratives. The American journal of drug and alcohol abuse, 47(4), 444–454